Identity Resolution 101 Part 1: Understanding the Fundamentals
Welcome to the first installment of our comprehensive series on Identity Resolution, aptly titled "Identity Resolution 101." As we navigate an increasingly complex digital ecosystem, understanding the foundational elements of identity resolution has never been more crucial. The techniques and tools that drive this intricate process have undergone seismic shifts in recent years, thanks to groundbreaking advancements in artificial intelligence and machine learning.
The field is no longer confined to basic data matching; it has evolved into a sophisticated mechanism that empowers businesses to construct a multi-dimensional view of their customers. Given these shifts, it's essential for marketers, data scientists, and even C-suite executives to recalibrate their understanding of how identity resolution works today, as opposed to a mere few years ago.
As a pioneer in leveraging advanced technologies to unravel the complexities of consumer data, Machintel is uniquely positioned to guide you through this labyrinth. Whether you're a seasoned pro looking to update your knowledge or a novice eager to grasp the basics, this series is designed to shed light on the indispensable facets of identity resolution, setting the stage for more advanced topics in subsequent installments.
What is Identity Resolution?
A Critical Tool for Understanding Your Customers
Identity Resolution isn't merely a buzzword in the tech world; it's a critical operational function that underpins successful customer relationship management, marketing strategies, and even cybersecurity measures. At its most fundamental level, Identity Resolution is the complex process by which businesses and organizations collect, analyze, and link fragmented customer data from myriad sources to construct a unified, comprehensive profile of an individual customer. In a digital landscape that's exponentially growing in complexity, Identity Resolution has proven to be indispensable.
Far from being a monolithic block, Identity Resolution is a confluence of several interdependent activities. These range from raw data collection from multiple customer touchpoints—such as mobile apps, websites, and even in-store interactions—to the application of intricate algorithms that cross-reference these fragmented pieces of information. The aim? To produce a cohesive and actionable portrait of your customer, also known as the Single Customer View (SCV).
Beyond Marketing: A Multi-Dimensional Utility
While marketing teams are perhaps the most frequent users of Identity Resolution, the practice extends far beyond the realm of advertising and customer outreach. Human resources departments, for example, leverage identity resolution techniques to create more comprehensive employee profiles. Security and fraud prevention sectors utilize it to identify unusual patterns of behavior that may signify a data breach or fraudulent activity. Financial services and healthcare, with their stringent regulatory demands, also employ identity resolution to ensure compliance and data accuracy.
The Pivotal Role of Identity Graphs
You might hear the term Identity Graph thrown around quite a bit in discussions about Identity Resolution. And for good reason: the Identity Graph is often the final output of a well-executed Identity Resolution process. It serves as a multidimensional roadmap, charting the relationships between different customer identifiers and painting a nuanced picture that's actionable across various business units.
Tools and Technologies
The rise of specialized Identity Resolution platforms has facilitated the automation of many of the steps involved in the process, incorporating machine learning algorithms for more accurate matching and data enrichment. These tools range from standalone software solutions to comprehensive platforms that integrate seamlessly with existing CRM or data management systems. While once the province of large enterprises, increasingly accessible tools now make Identity Resolution feasible for small to medium businesses as well.
An End-to-End Process
Regardless of what tools and techniques you use, the identity resolution process tends to follow the same 5 steps:
- Data Collection: Gathering disparate pieces of customer data from various touchpoints—think social media interactions, e-commerce shopping carts, or CRM databases.
- Data Normalization: Standardizing the collected data to create a consistent format, making it easier to work with.
- Identity Matching: Linking data attributes like email addresses, device IDs, or cookies to a single individual or entity.
- Identity Enrichment: Supplementing existing profiles with additional data for a more comprehensive understanding of the customer.
- ID Graph Construction: Creating an Identity Graph is often the end game. It's a data structure that maps out the relationships between different identifiers, offering a bird's-eye view of the customer.
Now that we've unpacked the complexities and broad applications of Identity Resolution, it's crucial to delve into why this process is becoming increasingly indispensable in today's digital landscape.
Why Identity Resolution Matters?
Solving Today's Digital Challenges
In our digitally interconnected landscape, brands and marketers are dealing with increasingly complex challenges. Chief among these is the problem of fragmented consumer data, gathered across multiple touchpoints from social media to in-store interactions. This data sprawl clouds the ability to form a comprehensive understanding of individual customer preferences, behaviors, and purchase history, thus making personalized engagement more difficult.
Organizations find themselves grappling with incomplete data that lacks essential information, making it hard to build a full picture of customer behaviors and needs. For example, a retail website may capture the types of products viewed but fail to capture why a potential customer abandoned a shopping cart.
The ever-changing nature of consumer preferences makes it essential for companies to keep their data updated. Stale or outdated data can be misleading and result in misguided strategies. A campaign based on last year's customer behaviors is no guarantee for success today.
The issue of data quality looms large over all of this. Minor inaccuracies can compound over time, leading to major issues in targeting and analytics. Companies must continually validate their data for accuracy to ensure its reliability as a business asset.
Finally, companies are under pressure to navigate a complicated web of compliance and data privacy regulations. Laws like GDPR in Europe and CCPA in California necessitate careful handling of consumer data. A properly implemented identity resolution strategy not only helps in unifying fragmented data but also ensures compliance with these stringent regulations.
Identity Resolution Use Cases: How Companies Are Benefiting
To better grasp the utility and indispensability of identity resolution, let's delve into some real-world use cases that demonstrate how companies are employing this technology to solve these challenges.
- Multi-Channel Marketing: Consolidated data can track customer interactions across multiple platforms, leading to more effective and personalized multi-channel marketing campaigns.
- Customer Retention: A comprehensive understanding of the customer journey can significantly improve customer satisfaction, thereby increasing retention rates.
- Fraud Prevention: Accurate identity resolution can be critical in identifying fraudulent activities, protecting both the company and its customers.
- Inventory Management: By understanding customer preferences at a granular level, companies can make more informed decisions about stock levels and product varieties.
- Behavioral Analytics: Deep insights into consumer behavior can guide more effective product development and marketing strategies.
The Stakes: What's at Risk?
The failure to invest in an effective identity resolution strategy is fraught with risks. Companies that fall behind can lose their competitive advantage, and it's not just about missing out on creating personalized experiences. In today's saturated digital environment—increasingly cluttered with AI-generated content—merely being visible is no longer sufficient. Consumers expect brands to not only recognize them but also understand their needs and preferences even before any direct engagement. This elevates the stakes for companies to intelligently cut through the noise and meaningfully connect with their audience.
Moreover, regulatory compliance becomes an even greater concern. Sloppy data management can result in significant legal consequences and hefty fines. On a broader scale, companies miss out on revenue-generating opportunities by lacking a unified, 360-degree view of their customer data.
Having delved into why identity resolution is not just a nice-to-have but a necessity in today’s complex digital landscape, let’s examine its historical evolution and how it has adapted to meet these modern challenges..
Having explored why identity resolution is critical in today's digital landscape, let’s take a look at where it comes from and how it’s evolved to meet these modern demands.
The Evolution of Modern Identity Matching Techniques
In the early days of digital marketing, businesses often relied on cookies to track user behavior across websites. While useful, these first-generation techniques were limited in scope. They couldn't offer a comprehensive view of the customer journey, especially across multiple devices or offline touchpoints.
Over time, the limitations of cookie-based approaches became apparent. Marketers needed a more unified, reliable method to resolve identities across various platforms and data sources. That led to the development of deterministic and probabilistic matching techniques.
Deterministic matching involves using known, concrete identifiers, like an email address, to link data across sources. Probabilistic matching, on the other hand, relies on statistical algorithms to make educated guesses about identity, using multiple data points like device type, location, and browsing behavior. These methods significantly improved identity resolution but still had limitations, particularly concerning scale and accuracy.
Enter the era of Artificial Intelligence and Machine Learning. These technologies have given identity resolution a significant boost. Advanced algorithms can now analyze vast sets of data in real-time, providing a much more nuanced and accurate view of customer identity. Moreover, AI-powered systems can adapt and improve, learning from new data to make better identity matches as they go along. This is where we stand today, in a significantly advanced stage of identity resolution, capable of providing a 360-degree customer view that's both scalable and precise.
Analysts have unanimously underscores the increasing sophistication and effectiveness of identity matching techniques, advocating for a combination of deterministic, probabilistic, and AI-driven methods for the most comprehensive and accurate identity resolution.
Next Steps
Machintel is at the forefront of this identity resolution revolution, providing cutting-edge solutions tailored to your business needs. Leveraging a blend of deterministic, probabilistic, and AI-driven techniques, we help companies navigate the complex landscape of identity data. Our platform goes beyond mere data collection; it turns fragmented, siloed information into a unified, actionable customer view. In a world where data drives decisions, partnering with Machintel means you're one step ahead in understanding your customers and exceeding their expectations.
In upcoming posts in this series, we'll dive deeper into the technicalities, applications, and ethical considerations of identity resolution. With Machintel as your guide, you'll gain the insights needed to navigate this complex yet crucial landscape.
Key Terms: An Identity Resolution Glossary
Understanding the terminology is a crucial step in mastering any subject matter, especially one as multifaceted as identity resolution. With a multitude of terms often used interchangeably or misunderstood, a glossary becomes an essential tool. Here's a list of key terms that will help you get a solid grasp on the concept.
- Address Normalization: The process of formatting and validating address data to ensure uniformity and accuracy.
- API (Application Programming Interface): A set of protocols for building and integrating application software.
- Attribute: Specific characteristics or properties that describe an entity within a data set.
- Audience Segmentation: Dividing a broad consumer or business market into sub-groups of consumers.
- Batch: A set of data or operations processed as a single unit, often at scheduled times.
- CCPA (California Consumer Privacy Act): California law aimed at enhancing privacy rights and consumer protection.
- CRM (Customer Relationship Management): Software for managing interactions with current and potential customers.
- Cross-Device Tracking: Monitoring user behavior across multiple devices.
- Data Deduplication: The elimination of duplicate or redundant data records.
- Data Enrichment: Enhancing existing information with additional data to make it more useful.
- Data Lake: A storage repository that holds a large amount of raw data in its native format until it is needed.
- Data Layer: A structural code layer that stores user data to be sent to various analytics tools.
- Data Onboarding: The process of transferring offline data online for digital processing and analysis.
- Data Record: A single unit of data stored in a database, containing attributes that represent various aspects of an entity.
- Data Silo: A repository of fixed data that an organization does not regularly use in its operational processes.
- Data Warehouse: A large, centralized repository of data that can be used for reporting and data analysis.
- Deterministic Matching: The use of unique identifiers to match customer data.
- Edge: In graph theory, a connection between two nodes that signifies a relationship between entities.
- Entity: A distinct object or concept in a data set, which can be identified by its attributes.
- Entity Fusion: The process of combining multiple related but distinct data records into a unified, single record.
- First-Party Data: Data collected directly from your audience or customers.
- Fuzzy Matching: A technique that finds approximate matches between data elements when exact matches are not available.
- GDPR (General Data Protection Regulation): European Union law on data protection and privacy.
- Geocoding: The process of converting addresses into geographic coordinates.
- Geomatching: Matching of geographical data based on location attributes.
- Golden Customer Record: A unified and validated customer profile that serves as the authoritative source of truth.
- ID Graph: A framework that connects multiple identifiers, across channels and devices, to one individual user.
- Identity Resolution: The process of matching disparate data points across multiple platforms to build a single, unified profile of an individual user.
- Multi-Touch Attribution: Assigning value to each customer interaction that led to a desired outcome.
- Node: A point within a network, often representing an entity, where connections intersect or branch.
- Omnichannel: A multi-channel sales approach that provides customers with an integrated customer experience.
- PII (Personally Identifiable Information): Information that can be used to uniquely identify an individual.
- Probabilistic Matching: The use of statistical algorithms to match customer data.
- Real-time: Processing and delivering data as it is created or received, without delay.
- Record Linkage: The practice of finding, matching, and linking records from different data sources that refer to the same entity.
- Retargeting: Online advertising that targets users who have previously interacted with a brand.
- Rules: Defined criteria or algorithms used to make decisions during the data matching process.
- SSO (Single Sign-On): An authentication process that allows a user to access multiple services with one set of credentials.
- Temporal Matching: The process of matching records based on time-based attributes.
- Third-Party Data: Data collected by an entity that does not have a direct relationship with the user the data is being collected on.
- Transformation: The process of converting data from one format or structure to another.
- Transitive Linking: A technique to infer relationships between entities that are both related to a common third entity but not directly to each other.
- Unified Customer Profile: A single view that combines all of a customer's interactions and engagements across channels.
- Cookie: A small file stored on a user's device that records browsing data.
Recent Posts

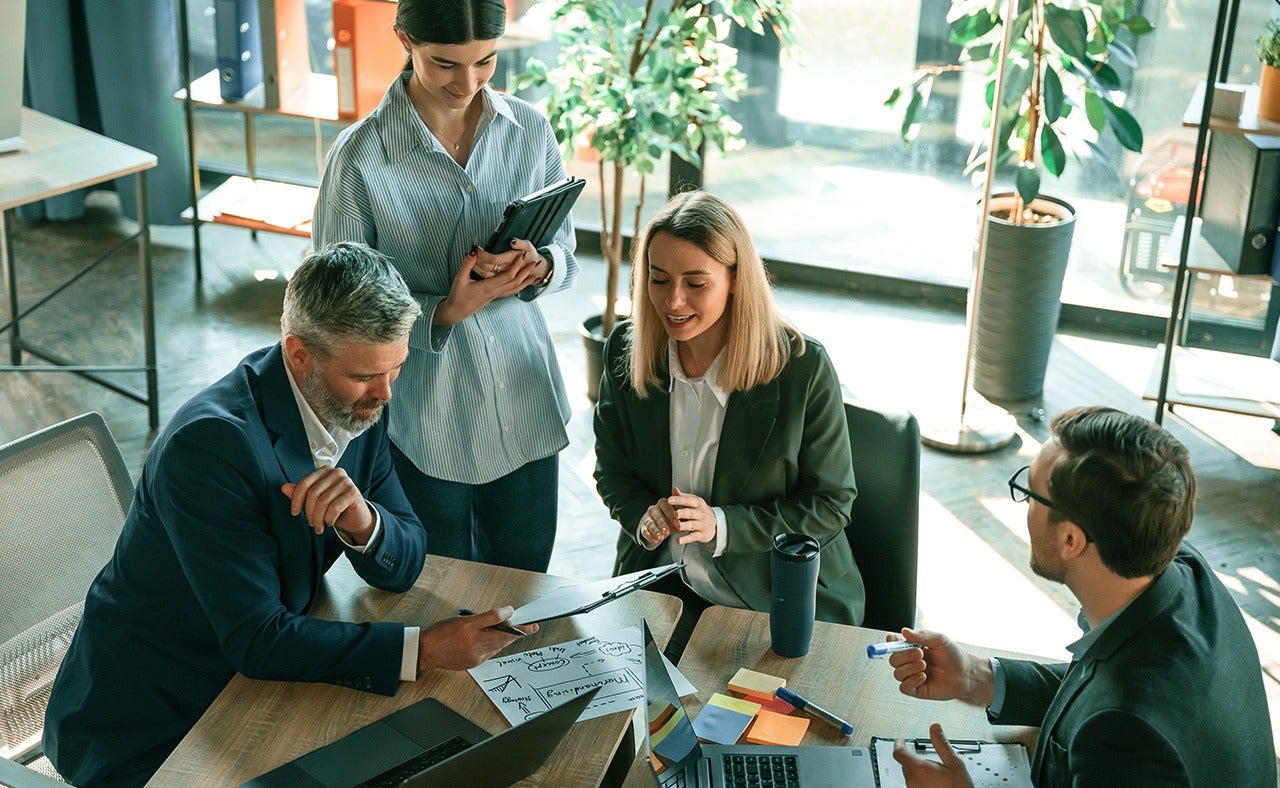
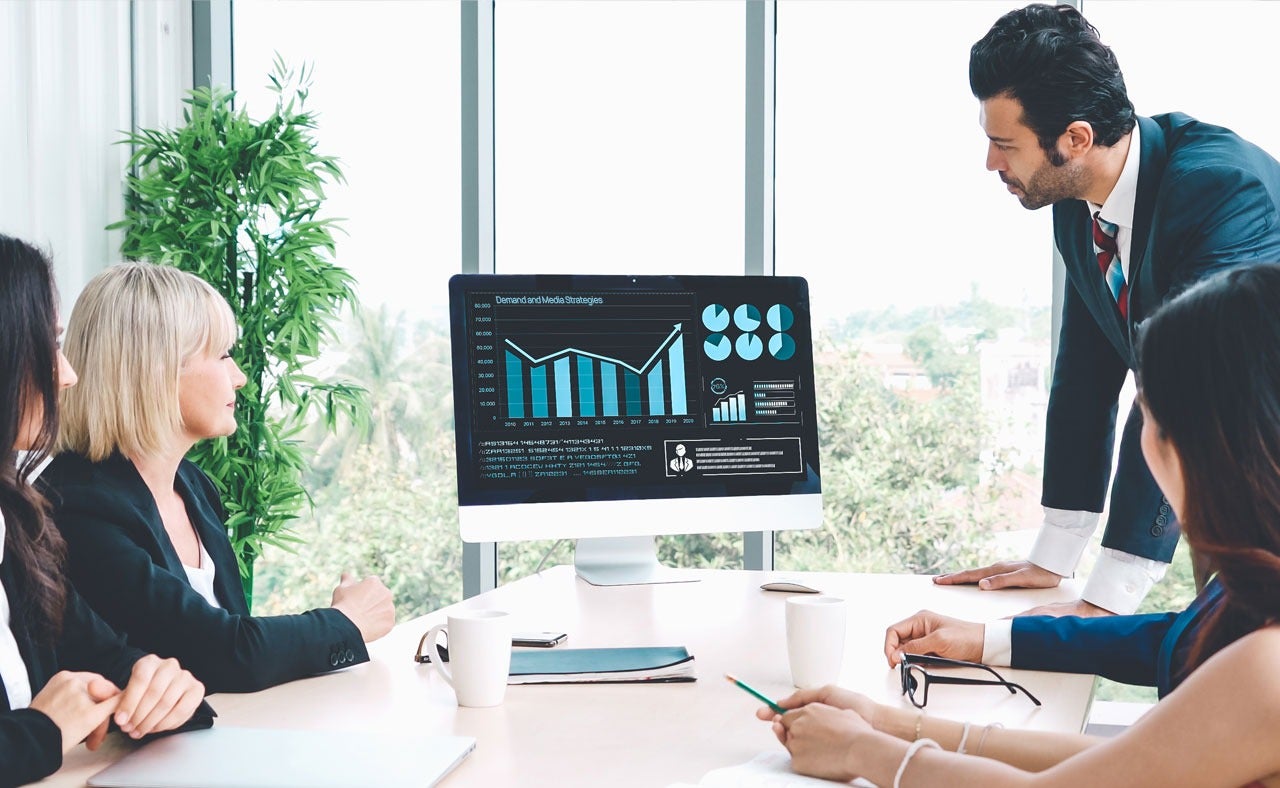
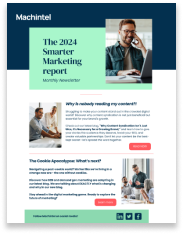
By submitting this form I have read and acknowledged the Terms of Use and the Privacy policy